Are Information and Data Lifecycle Management Processes Different…and Who Cares?
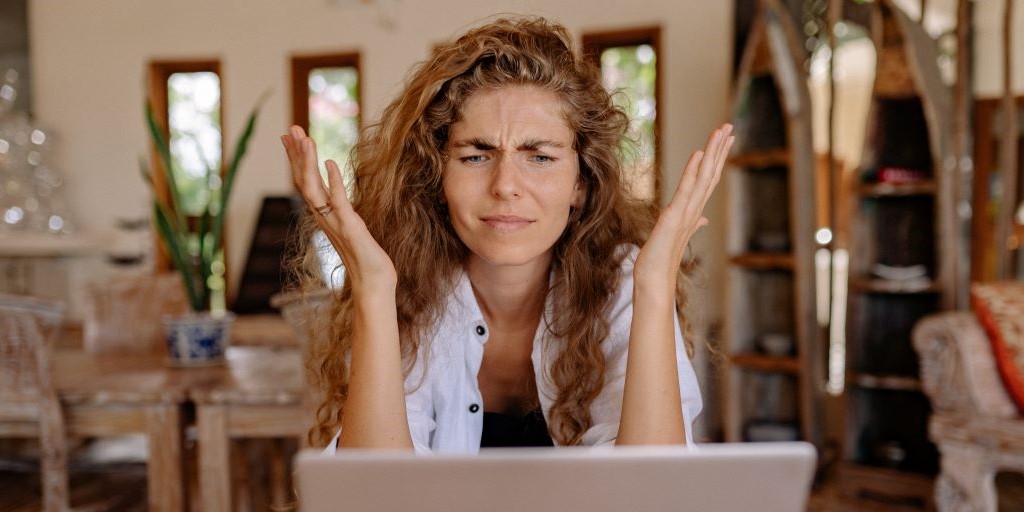
Are Data Lifecycle Management (DLM) and Information Lifecycle Management (ILM) just fancy terms for the exact same thing – namely, data management? Will understanding these terms actually impact your data strategy in any meaningful way? Will knowing this distinction affect your business at all?
Recently, we began a series of posts on data strategy by asking whether your company data is an asset or a utility. We then uncovered 10 Data Storage Considerations to Improve Your Company Data Strategy. In this post, I will argue that recognizing the distinction between DLM and ILM is important for IT leaders to help their companies get the most out of their data.
Now, the difference between Data Lifecycle Management (DLM) and Information Lifecycle Management (ILM) might initially seem like hair-splitting. However, while DLM incorporates management policies for the physical aspects of data as data (type, size, location, age, etc.), ILM addresses the content of the data as information (its accuracy, reliability, sensitivity/confidentiality, etc.).
Here are some of the differences and why both are important to consider.
Data Lifecycle Management
At its highest level, DLM addresses data creation, data management, and data deletion.
Within these categories, there are many other elements of data management that are included as well:
- Creation / Collection
- Classification
- Redundancy
- Duplication
- Integrity
- Usage and Availability
- Sharing
- Storage
- Security
- Archiving
All these data process elements when viewed through DLM are fundamentally concerned with the physical aspects of managing data. Failing to address these areas in your data strategy is to gamble with the health of your data structures and the value of your company data.
Information Lifecycle Management
On the other hand, ILM addresses issues associated with the information the data contains. ILM establishes policies that manage the data quality, business relevance, regulatory compliance, and legal liability of the data.
Many of the specific areas addressed by ILM establish protocols for processing data in a way that ensures data accuracy, reliable delivery, protection of sensitive information, and compliance with data privacy laws. Some elements of ILM can include:
- Data De-Identification / Masking
- Data Quality Frameworks & Audits
- Development and QA Environment Refreshes
- Source Control
- Master Data Management
- Classification and Governance
- Sharing & Usage
- Security
- Regulatory Compliance Audits
While many elements of the data lifecycle have relevance in both management models, they are viewed through a different lens in each model.
So…Who Cares?
So, why does recognizing this distinction matter? Will your business really suffer without it?
The answer is that it matters because if this distinction is not recognized, the two sets of management policies can get conflated, with one getting largely ignored. When either DLM or ILM is neglected, important elements of the data lifecycle can appear to be fully managed when in fact they are only partially addressed.
Perhaps even more importantly, when IT leaders do not see these management policies as distinct, they risk missing out on the benefits that come with using both together, like:
- Improved system performance
- Increased availability and accessibility of data
- Improved data quality
- Increased data consistency across the organization
- Improved recoverability
- Increased security
- Increased user satisfaction
- Controlled costs
- Improved regulatory compliance
In upcoming posts, we will unpack these models further while exploring more ways to improve your data strategy.
Want to work with The SERO Group?
Would you like some outside input in any of these areas? We love to work alongside IT leaders and their teams to help them establish the use of best practices in their data environments.
If that’s something you’d like to learn more about, let’s have a conversation.